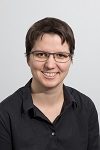
Extraordinary Associate Professor
Short Bio
Prof Lidia Auret is the Data Scientist and Process Manager at Stone Three Digital (a digital productivity and workplace safety solutions provider) and an Extraordinary Associate Professor at the Department of Process Engineering at Stellenbosch University.
Lidia’s research interest is data analytics and machine learning methods applied to the challenge of process monitoring in industrial processes, specifically in mineral processing systems. Through collaborating with industrial funders (e.g. Anglo American Platinum, Glencore) and industry-state partnerships (e.g. SAMMRI – South African Mines to Metals Research Initiative), her research has focussed on the application of data analytics to industrial processes – a vital component of Industry 4.0 technologies.
Prof Auret is the author of 40 peer-reviewed publications, including papers in leading international journals such as Journal of Process Control and Chemometrics and Intelligent Laboratory Systems, as well as peer-reviewed international conference contributions, including for IEEE (Institute of Electrical and Electronics Engineers) and IFAC (International Federation of Automatic Control) conferences. Lidia has successfully mentored one postdoctoral fellow, supervised 2 PhD and 16 master’s students, and currently supervises 2 PhD and 7 master’s students.
Lidia is a member of the Southern African Institute of Mining and Metallurgy and the South African Statistical Association. She serves on the executive committee of the South African Council for Automation and Control. Lidia is the co-chair of the national organizing committee for the 18th IFAC Symposium on Control, Optimization and Automation in Mining, Mineral and Metal Processing. She also serves as reviewer for established journals like Control Engineering Practice, Minerals Engineering, and Journal of Southern African Institute of Mining and Metallurgy.
Research Interests
Overall theme: Process monitoring with applications in extractive metallurgy / mineral processing
- Unsupervised machine learning techniques (random forests, artificial neural networks, kernel methods) as applied to process monitoring
- Validation of statistical process monitoring techniques by means of real-world fault libraries and dynamic simulation of process systems
- Enhancing process monitoring techniques by explicit inclusion of process knowledge (e.g. fundamental process model knowledge as well as fault type information inclusion through Bayesian networks and inference; post priori alarm classification knowledge inclusion for adaptive updating of process monitoring frameworks)
- Optimal sensor location techniques based on data reconciliation methods
- Computer vision applications in mineral processing systems (e.g. spiral concentrator interface detection, deep learning for froth flotation feature extraction)
- Economic performance analysis concepts applied to process monitoring frameworks
- Cost-benefit analysis of process monitoring, with specific application to mineral processing industries
Selected Publications
A complete list of her published works can be found on her Google Scholar profile.